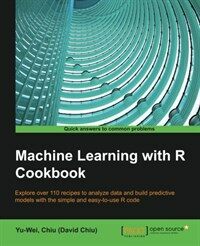
Machine learning with R cookbook: explore over 110 recipes to analyze data and build predictive models with the simple and easy-to-use R code
- 서명/저자사항
- Machine learning with R cookbook: explore over 110 recipes to analyze data and build predictive models with the simple and easy-to-use R code
- 개인저자
- Chiu, Yu-Wei
- 발행사항
- Birmingham : Packt, 2015.
- 형태사항
- xii, 430 p. : ill. ; 24 cm.
- ISBN
- 9781783982042 (pbk.)
- 주기사항
- Includes index
소장정보
위치 | 등록번호 | 청구기호 / 출력 | 상태 | 반납예정일 |
---|---|---|---|---|
이용 가능 (1) | ||||
자료실 | WM020571 | 대출가능 | - |
- 등록번호
- WM020571
- 상태/반납예정일
- 대출가능
- -
- 위치/청구기호(출력)
- 자료실
책 소개
Explore over 110 recipes to analyze data and build predictive models with the simple and easy-to-use R code
About This Book
- Apply R to simplify predictive modeling with short and simple code
- Use machine learning to solve problems ranging from small to big data
- Build a training and testing dataset from the churn dataset,applying different classification methods.
Who This Book Is For
If you want to learn how to use R for machine learning and gain insights from your data, then this book is ideal for you. Regardless of your level of experience, this book covers the basics of applying R to machine learning through to advanced techniques. While it is helpful if you are familiar with basic programming or machine learning concepts, you do not require prior experience to benefit from this book.
In Detail
The R language is a powerful open source functional programming language. At its core, R is a statistical programming language that provides impressive tools to analyze data and create high-level graphics.
This book covers the basics of R by setting up a user-friendly programming environment and performing data ETL in R. Data exploration examples are provided that demonstrate how powerful data visualization and machine learning is in discovering hidden relationships. You will then dive into important machine learning topics, including data classification, regression, clustering, association rule mining, and dimension reduction.