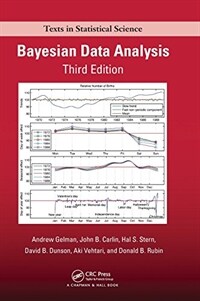
Bayesian data analysis
- 서명/저자사항
- Bayesian data analysis / John B. Carlin , Hal S. Stern , David B. Dunson , Aki Vehtari , Donald B. Rubin
- 판사항
- Third edition
- 개인저자
- Gelman, Andrew | Carlin, John B | Dunson, David B | Rubin, Donald B | Stern, Hal S | Vehtari, Aki
- 발행사항
- Boca Raton : CRC Press, 2014
- 형태사항
- xiv, 661 pages : illustrations ; 26cm.
- ISBN
- 9781439840955 (hardback)
- 주기사항
- Includes bibliographical references (pages 607-639) and indexes
소장정보
위치 | 등록번호 | 청구기호 / 출력 | 상태 | 반납예정일 |
---|---|---|---|---|
이용 가능 (1) | ||||
자료실 | WM020984 | 대출가능 | - |
- 등록번호
- WM020984
- 상태/반납예정일
- 대출가능
- -
- 위치/청구기호(출력)
- 자료실
책 소개
Winner of the 2016 De Groot Prize from the International Society for Bayesian Analysis
Now in its third edition, this classic book is widely considered the leading text on Bayesian methods, lauded for its accessible, practical approach to analyzing data and solving research problems. Bayesian Data Analysis, Third Edition continues to take an applied approach to analysis using up-to-date Bayesian methods. The authors?all leaders in the statistics community?introduce basic concepts from a data-analytic perspective before presenting advanced methods. Throughout the text, numerous worked examples drawn from real applications and research emphasize the use of Bayesian inference in practice.
New to the Third Edition
- Four new chapters on nonparametric modeling
- Coverage of weakly informative priors and boundary-avoiding priors
- Updated discussion of cross-validation and predictive information criteria
- Improved convergence monitoring and effective sample size calculations for iterative simulation
- Presentations of Hamiltonian Monte Carlo, variational Bayes, and expectation propagation
- New and revised software code
The book can be used in three different ways. For undergraduate students, it introduces Bayesian inference starting from first principles. For graduate students, the text presents effective current approaches to Bayesian modeling and computation in statistics and related fields. For researchers, it provides an assortment of Bayesian methods in applied statistics. Additional materials, including data sets used in the examples, solutions to selected exercises, and software instructions, are available on the book’s web page.
Winner of the 2016 De Groot Prize from the International Society for Bayesian Analysis
Now in its third edition, this classic book continues to take an applied approach to analysis using up-to-date Bayesian methods. Along with new and revised software code, this edition includes four new chapters on nonparametric modeling, updates the discussion of cross-validation and predictive information criteria, and improves convergence monitoring and effective sample size calculations for iterative simulation. It also covers weakly informative priors, boundary-avoiding priors, Hamiltonian Monte Carlo, variational Bayes, and expectation propagation. Data sets and other materials are available online.
목차
FUNDAMENTALS OF BAYESIAN INFERENCE
Probability and Inference
Single-Parameter Models
Introduction to Multiparameter Models
Asymptotics and Connections to Non-Bayesian Approaches
Hierarchical Models
FUNDAMENTALS OF BAYESIAN DATA ANALYSIS
Model Checking
Evaluating, Comparing, and Expanding Models
Modeling Accounting for Data Collection
Decision Analysis
ADVANCED COMPUTATION
Introduction to Bayesian Computation
Basics of Markov Chain Simulation
Computationally Efficient Markov Chain Simulation
Modal and Distributional Approximations
REGRESSION MODELS
Introduction to Regression Models
Hierarchical Linear Models
Generalized Linear Models
Models for Robust Inference
Models for Missing Data
NONLINEAR AND NONPARAMETRIC MODELS
Parametric Nonlinear Models
Basic Function Models
Gaussian Process Models
Finite Mixture Models
Dirichlet Process Models
APPENDICES
A: Standard Probability Distributions
B: Outline of Proofs of Asymptotic Theorems
C: Computation in R and Stan
Bibliographic Notes and Exercises appear at the end of each chapter.