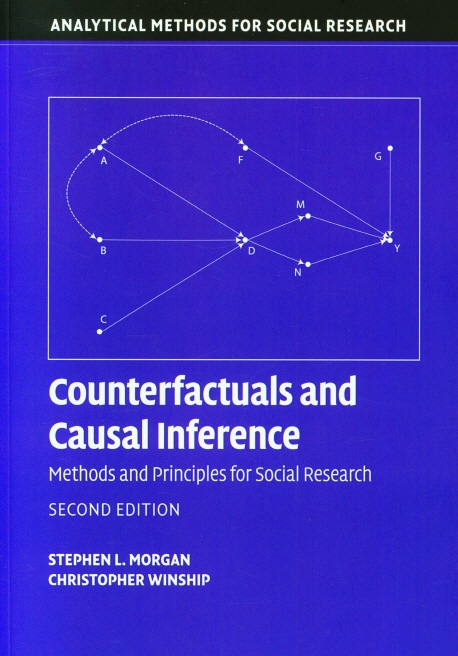
Counterfactuals and causal inference: methods and principles for social research
- 서명/저자사항
- Counterfactuals and causal inference : methods and principles for social research
- 개인저자
- Morgan, Stephen L 1971- | Winship, Christopher
- 발행사항
- New York, NY : Cambridge University Press, 2015.
- 형태사항
- xxiv, 499 p. ; 25 cm.
- ISBN
- 9781107694163
- 주기사항
- Revised edition of the authors' Counterfactuals and causal inference, published in 2007 Includes bibliographical references and index
소장정보
위치 | 등록번호 | 청구기호 / 출력 | 상태 | 반납예정일 |
---|---|---|---|---|
이용 가능 (1) | ||||
자료실 | WM021108 | 대출가능 | - |
- 등록번호
- WM021108
- 상태/반납예정일
- 대출가능
- -
- 위치/청구기호(출력)
- 자료실
책 소개
This new edition aims to convince social scientists to take a counterfactual approach to the core questions of their fields.
목차
Part I. Causality and Empirical Research in the Social Sciences: 1. Introduction; Part II. Counterfactuals, Potential Outcomes, and Causal Graphs: 2. Counterfactuals and the potential-outcome model; 3. Causal graphs; Part III. Estimating Causal Effects by Conditioning on Observed Variables to Block Backdoor Paths: 4. Models of causal exposure and identification criteria for conditioning estimators; 5. Matching estimators of causal effects; 6. Regression estimators of causal effects; 7. Weighted regression estimators of causal effects; Part IV. Estimating Causal Effects When Backdoor Conditioning Is Ineffective: 8. Self-selection, heterogeneity, and causal graphs; 9. Instrumental-variable estimators of causal effects; 10. Mechanisms and causal explanation; 11. Repeated observations and the estimation of causal effects; Part V. Estimation When Causal Effects Are Not Point Identified by Observables: 12. Distributional assumptions, set identification, and sensitivity analysis; Part VI. Conclusions: 13. Counterfactuals and the future of empirical research in observational social science.