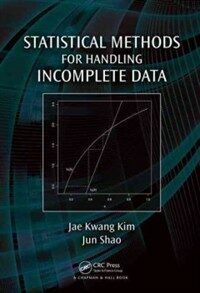
Statistical methods for handling incomplete data
- 서명/저자사항
- Statistical methods for handling incomplete data
- 발행사항
- Boca Raton : CRC Press, 2013.
- 형태사항
- xi, 211 p. ; 25 cm
- ISBN
- 9781439849637 (hardback : acid-free paper)
- 주기사항
- "With the advances in statistical computing, there has been a rapid development of techniques and applications in missing data analysis. This book aims to cover the most up-to-date statistical theories and computational methods for analyzing incomplete data through (1)vigorous treatment of statistical theories on likelihood-based inference with missing data, (2) comprehensive treatment of computational techniques and theories on imputation, and (3) most up-to-date treatment of methodologies involving propensity score weighting, nonignorable missing, longitudinal missing, survey sampling application, and statistical matching. The book is suitable for use as a textbook for a graduate course in statistics departments or as a reference book for those interested in this area. Some of the research ideas introduced in the book can be developed further for specific applications"--Provided by publisher Includes bibliographical references and index
소장정보
위치 | 등록번호 | 청구기호 / 출력 | 상태 | 반납예정일 |
---|---|---|---|---|
이용 가능 (1) | ||||
자료실 | WM019716 | 대출가능 | - |
- 등록번호
- WM019716
- 상태/반납예정일
- 대출가능
- -
- 위치/청구기호(출력)
- 자료실
책 소개
목차
IntroductionIntroduction Outline How to Use This Book Likelihood-Based ApproachIntroductionObserved LikelihoodMean Score ApproachObserved Information Computation IntroductionFactoring Likelihood ApproachEM AlgorithmMonte Carlo Computation Monte Carlo EM Data Augmentation ImputationIntroductionBasic Theory for ImputationVariance Estimation after Imputation Replication Variance EstimationMultiple ImputationFractional Imputation Propensity Scoring Approach Introduction Regression Weighting Method Propensity Score Method Optimal Estimation Doubly Robust Method Empirical Likelihood Method Nonparametric Method Nonignorable Missing DataNonresponse Instrument Conditional Likelihood Approach Generalized Method of Moments (GMM) Approach Pseudo Likelihood Approach Exponential Tilting (ET) Model Latent Variable Approach Callbacks Capture?Recapture (CR) Experiment Longitudinal and Clustered DataIgnorable Missing Data Nonignorable Monotone Missing DataPast-Value-Dependent Missing DataRandom-Effect-Dependent Missing Data Application to Survey Sampling Introduction Calibration Estimation Propensity Score Weighting Method Fractional Imputation Fractional Hot Deck Imputation Imputation for Two-Phase Sampling Synthetic Imputation Statistical Matching Introduction Instrumental Variable Approach Measurement Error ModelsCausal Inference Bibliography Index