Research Monographs
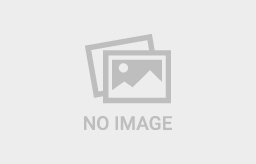
A Study of Quality Improvement in Health and Welfare Panel Data - Focusing on Imputation of Item Nonresponse
- Author
Lee, Hyejung
- Publication Date
2019
- Pages
- Series No.
- Language
Panel data is widely used in social, natural, healthcare and medical sciences, as it enables dynamic analysis of inter-subject differences and changes over time in the same subject. As in other forms of data, nonresponse in panel surveys occurs when the observed values for some variables are not measured. Nonresponse can be attributed to lack of bond at the beginning of the survey and increased panel fatigue as the investigation progresses. Imputation of missing responses is necessary to improve data quality.
This study is about bringing quality improvement to KOWEPS and KHP data, especially in terms of imputation of item nonresponse. We examined how Korea and other countries have been handling missing reponses in panel data. Also, machine learning and deep learning techniques were examined as a potential alternative to imputation of missing responses in panel data. Our simulation results show that imputation methods based on machine learning and deep learning generally outperform mean and hot-deck imputation. Specifically, we propose an imputation method based on random forest. It has been found that a large number of explanatory variables do not necessarily improve performance. Exploring and selecting variables that are highly related to the target imputation variables perform better than using a complex and comprehensive model.
Panel data is widely used as data for policy diagnosis and establishment. Imputation of item nonresponse is an important part of data quality management and must be managed continuously for statically reliable data production.
Attachments
- 첨부파일
Research Paper(수시)+2019-08+A Study of Quality Improvement ~.pdf